Note
Click here to download the full example code
Comparison of methods for correcting p-values for multiple comparisons¶
This script illustrates three methods to correct the p-values for multiple comparisons (i.e by the number of phases and by the number of amplitudes) :
Using the maximum statistics
Using a FDR correction
Using a Bonferroni correction
Note that for the FDR and Bonferroni corrections, MNE-Python is needed.
import numpy as np
from tensorpac import Pac
from tensorpac.signals import pac_signals_wavelet
import matplotlib.pyplot as plt
Simulate artificial coupling¶
first, we generate several trials that contains a coupling between a 6z phase and a 90hz amplitude. By default, the returned dataset is organized as (n_epochs, n_times) where n_times is the number of time points and n_epochs is the number of trials
f_pha = 6 # frequency phase for the coupling
f_amp = 90 # frequency amplitude for the coupling
n_epochs = 30 # number of trials
n_times = 4000 # number of time points
sf = 512. # sampling frequency
data, time = pac_signals_wavelet(f_pha=f_pha, f_amp=f_amp, noise=.4,
n_epochs=n_epochs, n_times=n_times, sf=sf)
Compute true PAC estimation and surrogates distribution¶
Now, we compute the PAC using multiple phases and amplitudes such as the distribution of surrogates. In this example, we used the method proposed by Tort et al. 2010 [11]. This method consists in swapping phase and amplitude trials. Then, we used the method
tensorpac.Pac.infer_pvalues
in order to get the corrected p-values across all possible (phase, amplitude) frequency pairs.
# define the Pac object
p = Pac(idpac=(1, 1, 0), f_pha='mres', f_amp='mres')
# compute true pac and surrogates
n_perm = 200 # number of permutations
xpac = p.filterfit(sf, data, n_perm=n_perm, n_jobs=-1).squeeze()
plt.figure(figsize=(16, 5))
for n_mcp, mcp in enumerate(['maxstat', 'fdr', 'bonferroni']):
# get the corrected p-values
pval = p.infer_pvalues(p=0.05, mcp=mcp)
# set to gray non significant p-values and in color significant values
pac_ns = xpac.copy()
pac_ns[pval <= .05] = np.nan
pac_s = xpac.copy()
pac_s[pval > .05] = np.nan
plt.subplot(1, 3, n_mcp + 1)
p.comodulogram(pac_ns, cmap='gray', colorbar=False, vmin=np.nanmin(pac_ns),
vmax=np.nanmax(pac_ns))
p.comodulogram(pac_s, title=f'MCP={mcp}', cmap='viridis',
vmin=np.nanmin(pac_s), vmax=np.nanmax(pac_s))
plt.gca().invert_yaxis()
plt.tight_layout()
p.show()
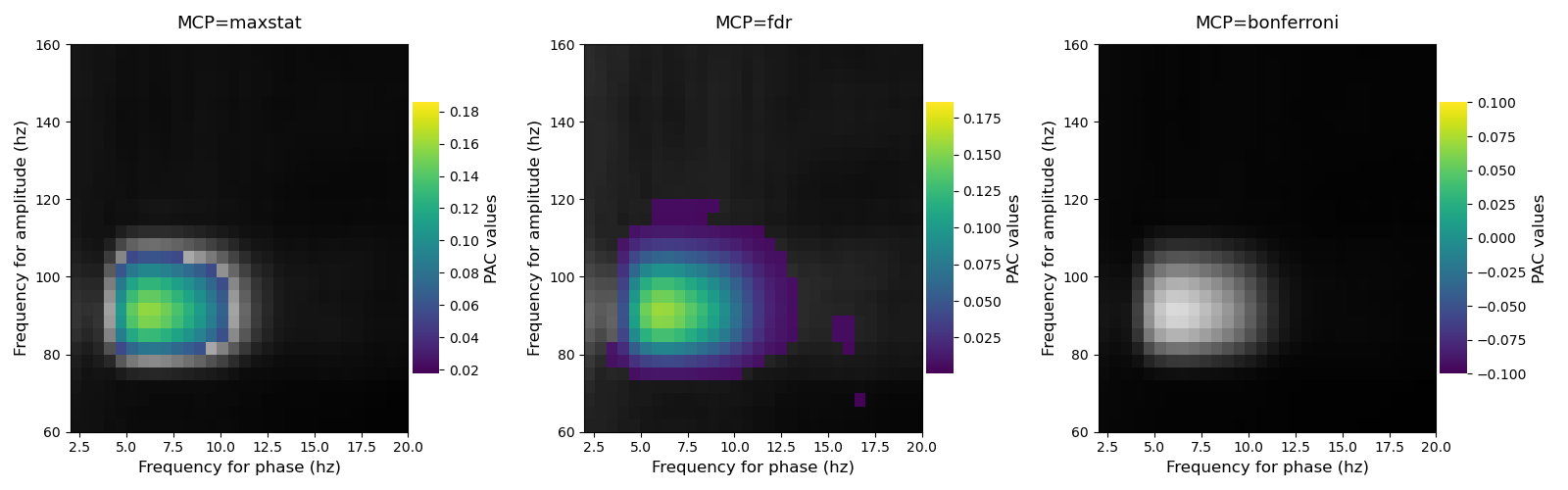
Out:
/home/circleci/project/examples/stats/plot_compare_mcp.py:69: RuntimeWarning: All-NaN slice encountered
vmin=np.nanmin(pac_s), vmax=np.nanmax(pac_s))
Total running time of the script: ( 0 minutes 17.890 seconds)