Research and highlights
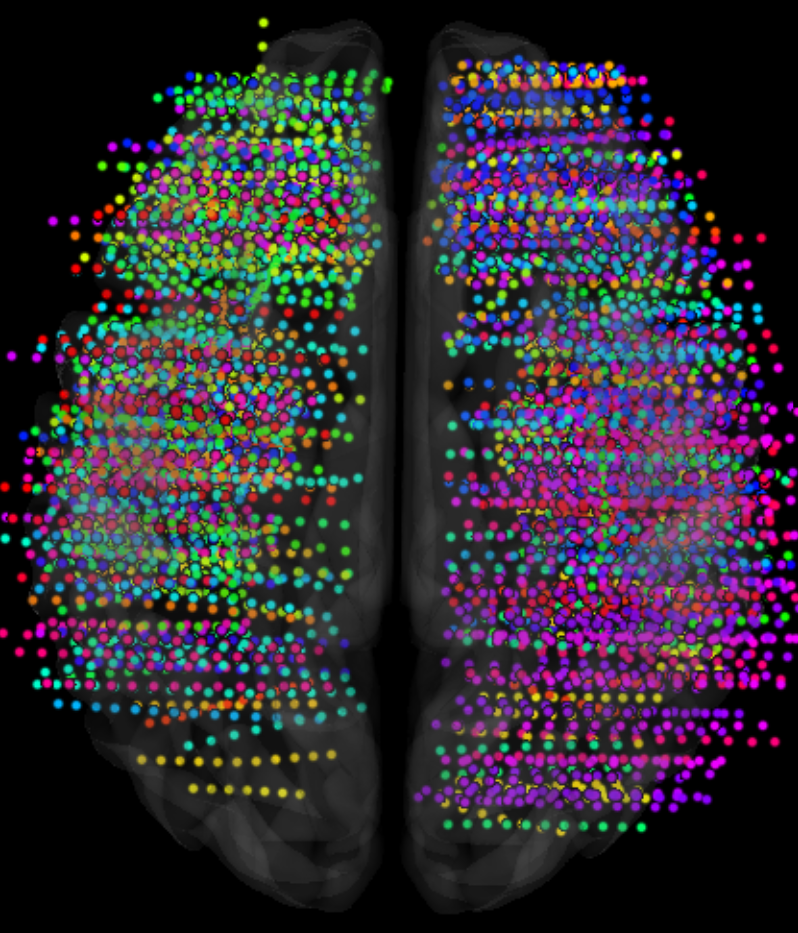
In the realm of brain research, some regions are known to carry out highly specialized tasks, particularly within sensory systems. Simultaneously, an increasing body of evidence suggests that different parts of the brain can perform similar cognitive functions, or at least yield comparable results. Moreover, a neural structure's function may depend on the larger network it is a part of. Adding to the complexity, there is significant variability among individuals, raising questions about the consistency of the relationship between brain structure and function.
My research endeavors to introduce cutting-edge open-source frameworks that shed light on integrative brain dynamics and their pivotal role in shaping complex human cognition. My approach involves harnessing the latest advances in statistics to precisely pinpoint how cognitive information is distributed and represented over time. Specifically, I aim to investigate how segregated computational processes dynamically come together during goal-oriented learning and decision-making, using neurophysiological recordings in humans.
To conduct this research, I employ a combination of machine-learning models and information-theoretic approaches. These methods help unravel what is computed locally versus what is distributed, shared, and complementary in the brain's intricate workings. Additionally, my growing interest in statistical inferences and group-level analyses stems from the belief that these filters play a crucial role in shaping our understanding of the brain.
Furthermore, I am deeply committed to open-source software and programming. I routinely package the methodologies utilized by our research team into concise Python packages. This not only enhances reproducibility but also empowers students and non-programming experts to pick up where my research leaves off, fostering a more collaborative and accessible research environment.